Few sentences about CSX-AI
CSX-AI is a timely project and start-up with the readiness from two perspectives. First, with the background that all major players in the world, such as the US, EU, UK, and China, are all developing their own AI regulations, technological support for companies developing AI products will be in high demand. Soon, it is expected that an AI product with safety and trustworthiness certification will be allowed to enter the market. The product and service CSX-AI offers will have a large, fast-growing market. Second, the technological support for safe and trusted AI products has a significant technical barrier, while the CSX-AI team is in a distinct position. Regulations require, instead of various tricks to be integrated into AI products, rigorous methods, well-grounded theories, and carefully engineered tools to evaluate and check whether the AI products satisfy desired safety and trustworthiness properties. The CSX-AI has been pioneering in this research direction, with seminal works and software tools available to be leveraged in the start-up. CSX-AI is technologically ready in an area with significant technological barriers.
More about us
Our History
CSX-AI was founded in 2024 by professors and researchers from top French and UK universities. The founders are top researchers in building correct, intelligent systems and have many years of R&D experience with successful software tools adopted by the market. We established CSX-AI to commercialize the research outcomes developed from numerous EU and national research projects, focusing mainly on rigorous techniques to ensure AI systems’ safety, security, and explainability.
CSX-AI has unique skills in these aspects, with the main advances in rigorous methods that provide provable guarantees. Rigorous methods are necessary for AI regulations as they can provide evidence of the satisfiability of the properties and enable traceability and accountability for failures. Our uniqueness is two-fold. On the one hand, our platform and tools support responsible development, ensuring that the final AI systems’ properties (e.g., robustness, fairness, privacy) are estimated and assured with errors bounded and traceable. On the other hand, our platform and tools can work efficiently with large-scale AI systems, minimizing the negative impacts of rigorous techniques that usually do not scale.
Our Team
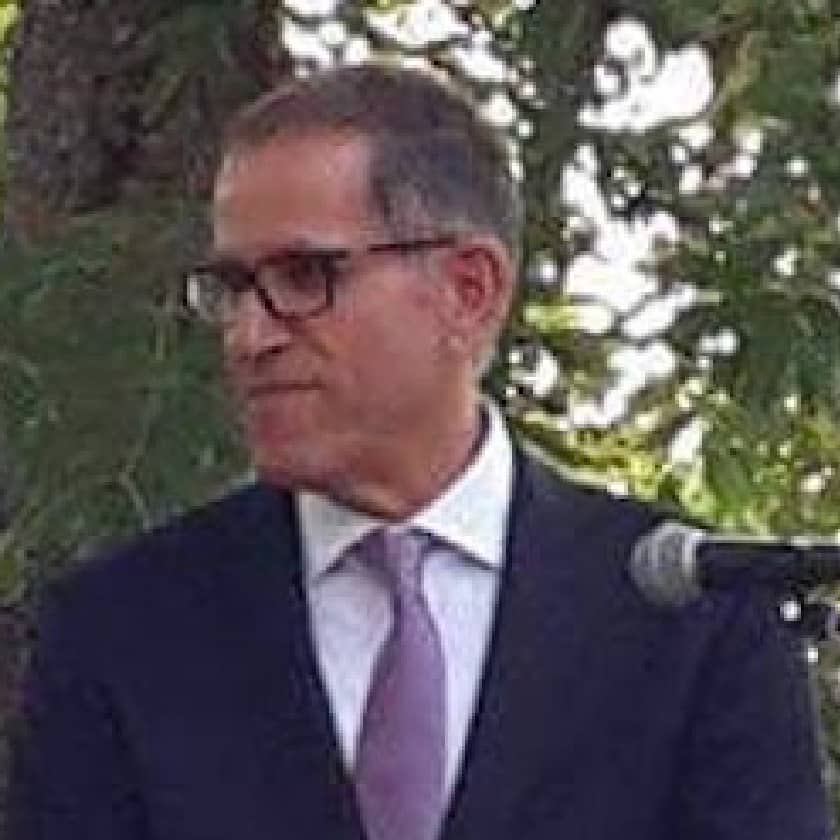
Saddek Bensalem
CEO Prof. University Grenoble Alpes (1986 - 2024)
He was the rigorous systems design team leader and recently the leader of the “Engineering of Trustworthy Learning-Enabled Systems” project. His research is concerned with developing automated verification techniques that ensure the correctness and reliability of Learning-Enabled Systems. He is leading the research direction on verifying and validating deep neural networks. He has published 200+ papers and was a PI for 30 national and EU projects.
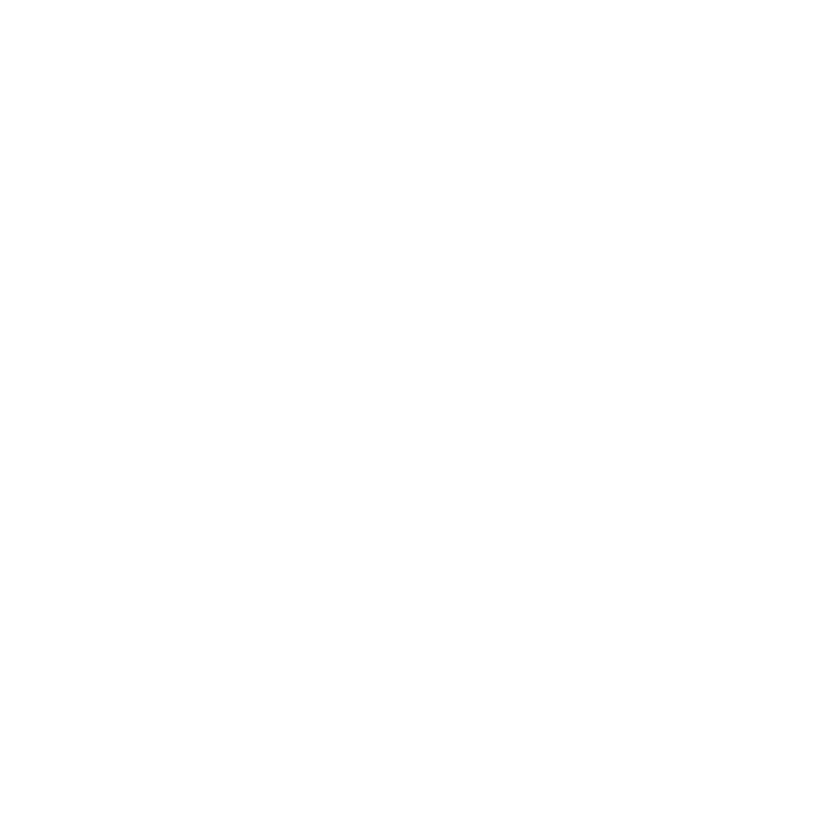
Changshun Wu
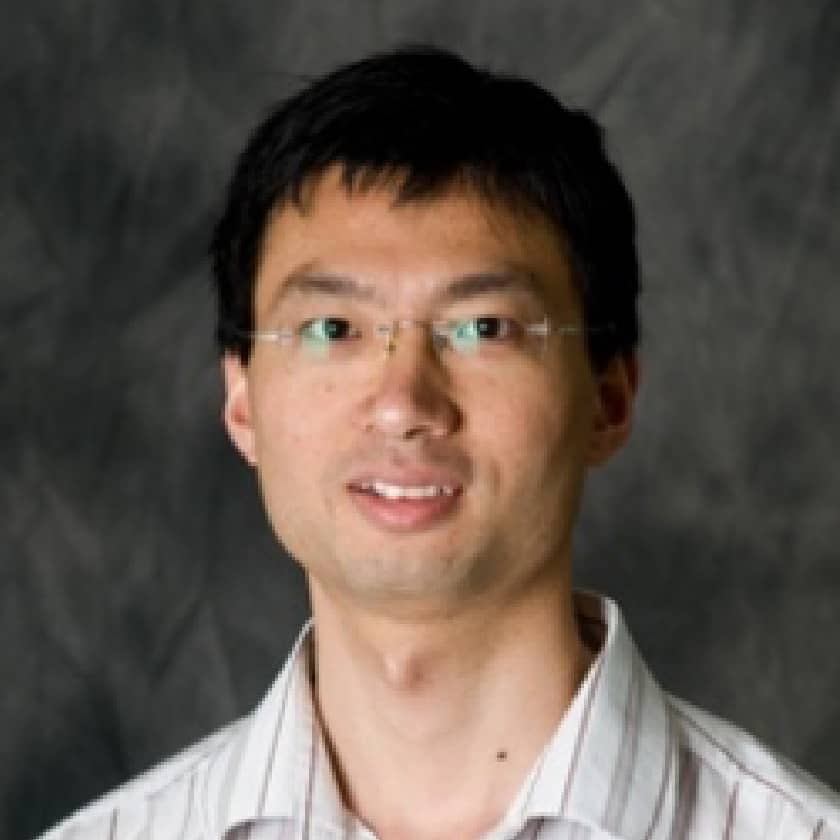
Xiaowei Huang
CSO Prof. University of Liverpool
He is the Director of the Trustworthy Autonomous CPS Lab. He is the Research Lead of the School of Electronic Engineering, Electronics, and Computer Science at the University of Liverpool (UoL). His research is concerned with developing automated verification techniques that ensure the correctness and reliability of intelligent systems. He is leading the research direction on verifying and validating deep neural networks. He authored a book <<Machine Learning Safety>> published by Springer in 2023, and has published 100+ papers.
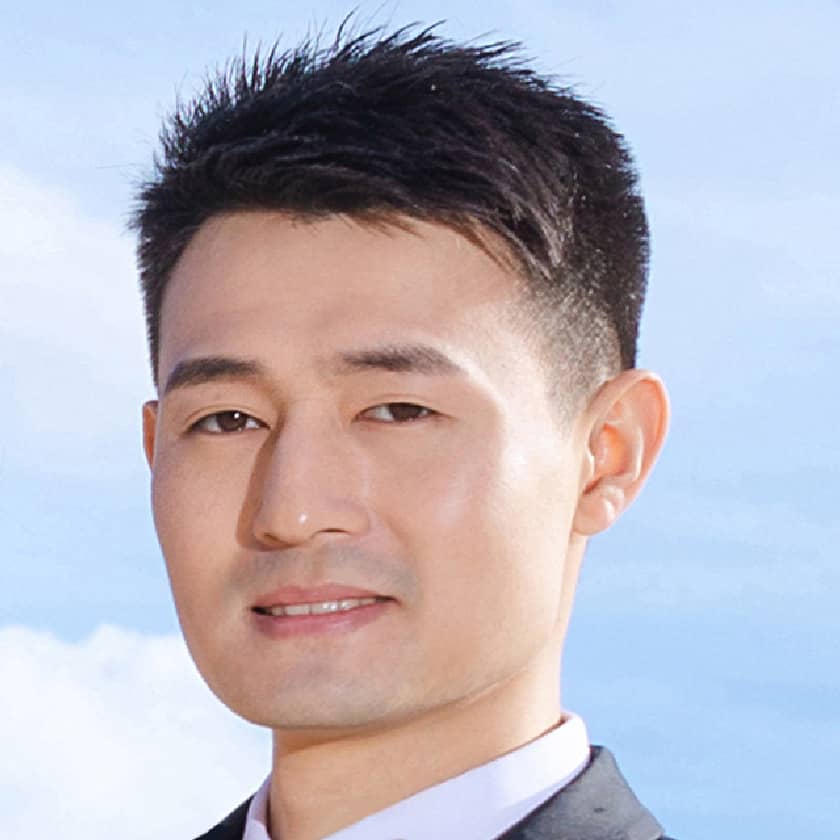
Dong Yi
He is an Assistant Professor at The University of Liverpool, specialising in Trustworthy AI with applications in robotics and energy systems. Dr. Dong also holds a visiting academic position at the University of Manchester. Prior to joining Liverpool, he was a New Frontiers Fellow at The University of Southampton. Dr. Dong has published over 20+ papers in the interdisciplinary fields of AI, robotics, and energy systems. His research interests include Trustworthy AI, Responsible AI, Safeguarding of LLMs, Distributed Optimisation, etc.